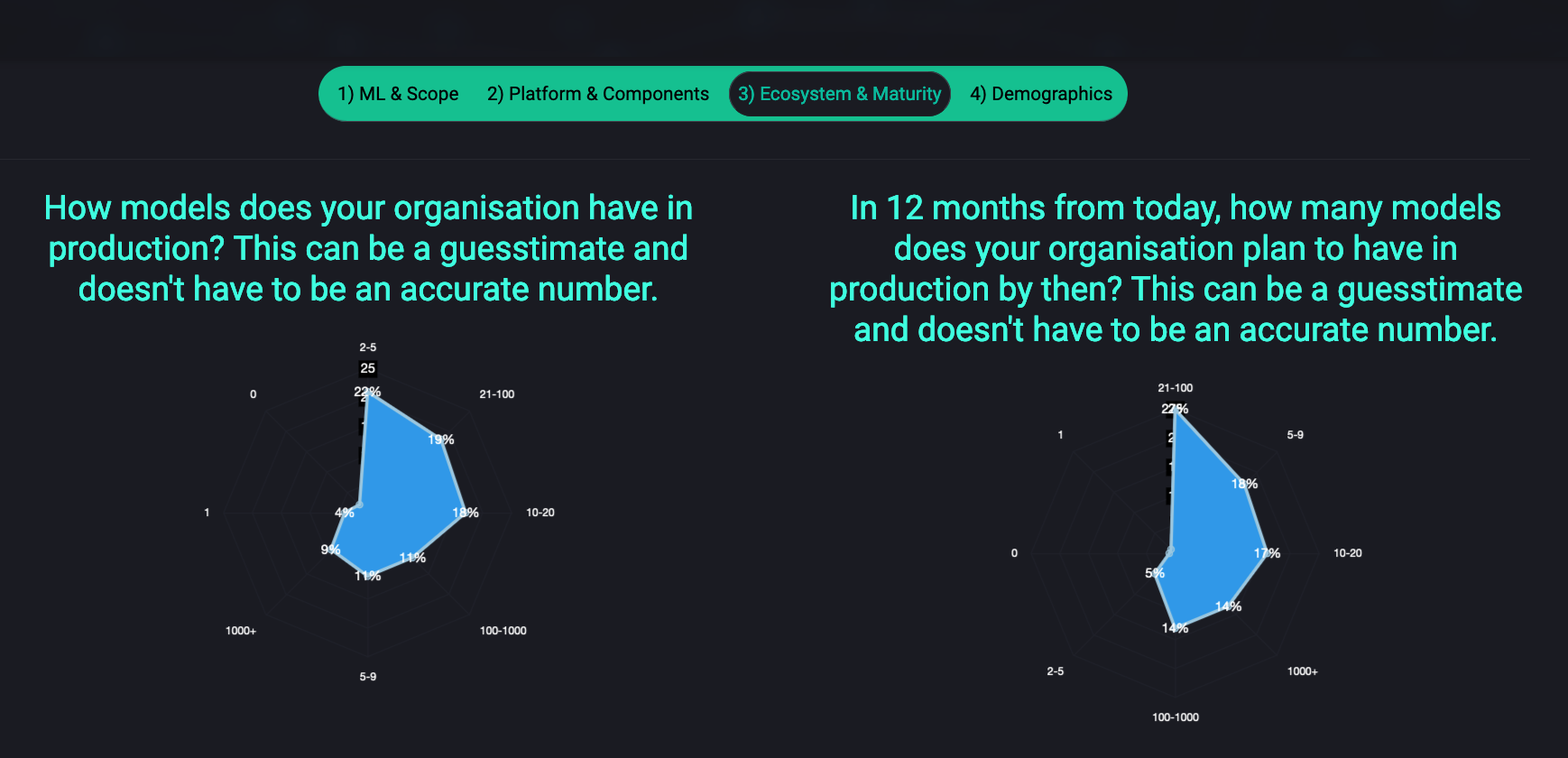 | Number of ML Models in Prod Almost 70% of teams reported to have less than 100 models in production; however most of them are looking to double the number of models in production within the next 12 months. This provides an interesting snapshot of the production ML ecosystem, as although many teams are operating at smaller relative sclaes, the rate of growth is only increasing; we can see this with 9-15% of teams operating already over 1000 models in production. We are uncovering important insights as part of our survey on The State of Production ML in 2024; please contribute to this valuable investigation on machine learning tools and platforms used in your production ML development. Your input will help create a comprehensive overview of common practices, tooling preferences, and challenges faced when deploying models to production, ultimately benefiting the entire ML community 🚀 |
|
|
---|
|
Building a GenAI Platform Chip Huyen has put together a fantastic resource on production architectural patterns and best practices for building GenAI platforms: This is a great guide for production machine learning practitioners on building a robust generative AI platform, starting from a basic model deployment and progressively adding essential components such as enhanced context with retrieval-augmented generation (RAG), safety guardrails to prevent data leakage and manage outputs, model routing and gateways for scalability and control, caching strategies for latency and cost optimization, and complex logic with write actions for advanced capabilities. This is a very much needed resource, as it emphasises the importance of observability and orchestration to monitor, debug, and manage complex AI pipelines effectively. |
|
|
---|
|
Open Source Agentic Workflows Our Production ML Github List has reached over 17,000+ stars 🚀 this provides a snapshot of open source tools in the ecosystem and we just added "Agentic Frameworks" to the list! We have put together this list to help machine learning practitioners to deploy, monitor, version, scale, and secure their production ML systems. The new "Agentic Workflow" section captures the tools available for building AI agents and multi-agent systems, including libraries like AgentScope, AutoGen, Chidori, LangGraph, between many others. If you know of any open source framework that is not listed please do give us a heads up or feel free to open up a PR! |
|
---|
|
Over 1000 Python Videos This is an absolutely fantastic to browse over 1000 python videos; more importantly for machine learning practitioners you can find a curated collection of machine learning talks from various conferences like PyCon, PyData, and EuroPython: This is quite a great resource for people that are looking to develop continuously, particularly in the ML space, there are a broad range of talks which cover topics such as scalable machine learning pipelines, MLOps best practices, model deployment strategies, deep learning advancements, and practical applications in industries like finance, healthcare, and technology. |
|
|
---|
|
Advent of Code 2024 The time has arrived to brush up our skills and jump into the advent of code. There will be one programming challenge released every day to take your skills to the test and have some fun, this is a great time to also pick up a new programming language if you've been wanting to explore one for a while. It's also interesting that different to previous years, this time there is a big disclaimer discouraging the use of LLMs for submissions due to the increasing use of these; it is interesting to see how these become more pervasive, and perhaps also how some of these type of challenges will also have to adapt through time to make it such that they can still be providing a challenge despite AI-supported development. |
|
|
---|
|
Upcoming MLOps Events The MLOps ecosystem continues to grow at break-neck speeds, making it ever harder for us as practitioners to stay up to date with relevant developments. A fantsatic way to keep on-top of relevant resources is through the great community and events that the MLOps and Production ML ecosystem offers. This is the reason why we have started curating a list of upcoming events in the space, which are outlined below. Upcoming conferences where we're speaking: Other upcoming MLOps conferences in 2024:
In case you missed our talks:
|
|
---|
| |
Check out the fast-growing ecosystem of production ML tools & frameworks at the github repository which has reached over 10,000 ⭐ github stars. We are currently looking for more libraries to add - if you know of any that are not listed, please let us know or feel free to add a PR. Four featured libraries in the GPU acceleration space are outlined below. - Kompute - Blazing fast, lightweight and mobile phone-enabled GPU compute framework optimized for advanced data processing usecases.
- CuPy - An implementation of NumPy-compatible multi-dimensional array on CUDA. CuPy consists of the core multi-dimensional array class, cupy.ndarray, and many functions on it.
- Jax - Composable transformations of Python+NumPy programs: differentiate, vectorize, JIT to GPU/TPU, and more
- CuDF - Built based on the Apache Arrow columnar memory format, cuDF is a GPU DataFrame library for loading, joining, aggregating, filtering, and otherwise manipulating data.
If you know of any open source and open community events that are not listed do give us a heads up so we can add them! |
|
---|
| |
As AI systems become more prevalent in society, we face bigger and tougher societal challenges. We have seen a large number of resources that aim to takle these challenges in the form of AI Guidelines, Principles, Ethics Frameworks, etc, however there are so many resources it is hard to navigate. Because of this we started an Open Source initiative that aims to map the ecosystem to make it simpler to navigate. You can find multiple principles in the repo - some examples include the following: - MLSecOps Top 10 Vulnerabilities - This is an initiative that aims to further the field of machine learning security by identifying the top 10 most common vulnerabiliites in the machine learning lifecycle as well as best practices.
- AI & Machine Learning 8 principles for Responsible ML - The Institute for Ethical AI & Machine Learning has put together 8 principles for responsible machine learning that are to be adopted by individuals and delivery teams designing, building and operating machine learning systems.
- An Evaluation of Guidelines - The Ethics of Ethics; A research paper that analyses multiple Ethics principles.
- ACM's Code of Ethics and Professional Conduct - This is the code of ethics that has been put together in 1992 by the Association for Computer Machinery and updated in 2018.
If you know of any guidelines that are not in the "Awesome AI Guidelines" list, please do give us a heads up or feel free to add a pull request!
|
|
---|
| |
| | The Institute for Ethical AI & Machine Learning is a European research centre that carries out world-class research into responsible machine learning. | | |
|
|
---|
|
|
This email was sent to You received this email because you are registered with The Institute for Ethical AI & Machine Learning's newsletter "The Machine Learning Engineer" |
| | |
|
|
---|
|
© 2023 The Institute for Ethical AI & Machine Learning |
|
---|
|
|
|