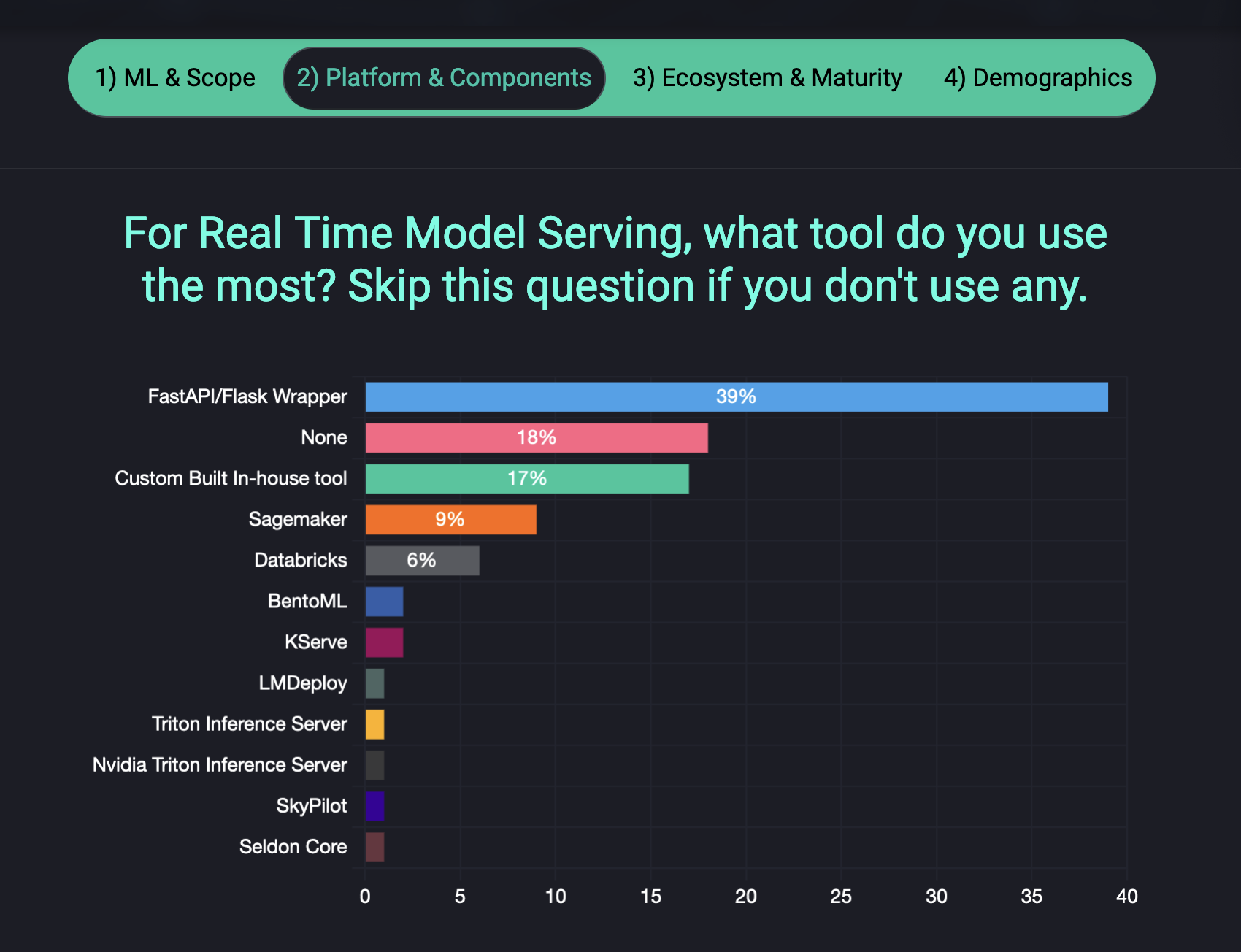 | State of Prod ML 2024 Survey Almost 60% of real-time machine learning is still powered by FastAPI/Flask or Custom Wrappers - it seems the MLOps ecosystem is not yet consolidated, showing huge opportunity in this space: These are really important insights from 2024 Survey on The State of Production ML; we have designed the questions to provide meaningful insights on the current landscape of production ML in 2024 - if you have a chance we would be grateful if you could spend a few minutes on the survey, as you'll contribute valuable information about the machine learning tools and platforms you use in your production ML development. Your input will help create a comprehensive overview of common practices, tooling preferences, and challenges faced when deploying models to production, ultimately benefiting the entire ML community 🚀 We are also working on an interactive visualisation for everyone to be able to slice and dice across the data to derive meaningful insights on the production ML ecosystem! |
|
|
---|
|
AirStreetCapital State of AI Report The State of AI Report 2024 from AirStreet Capital is out! Really interesting insights from 2024, including highlights on the performance gap between proprietary models like GPT-4 and open-source alternatives has narrowed significantly, with advancements in planning, reasoning, and multimodal capabilities extending AI's applications into fields like mathematics, biology, and neuroscience. One interesting observation is that despite U.S. sanctions, Chinese labs continue to produce competitive AI models through alternative means. Similarly, the economic impact of global AI has surged, with public companies reaching a combined enterprise value of $9 trillion, although questions about long-term sustainability and viable business models remain. Check out the full insights on the report! |
|
|
---|
|
Real Time Data Infra at Uber Uber has built a robust real-time data infrastructure to process Petabyte-scale data per day, using open-source technologies like Apache Kafka, Flink and Apache Pinot - these support production machine learning applications that require processing massive data volumes with low latency. Uber enables scalable stream processing and low-latency analytics critical for ML workflows like real-time prediction monitoring by enhancing these tools through leveraging frameworks such as FlinkSQL for easier streaming job creation with SQL and adding upsert capabilities to Pinot for real-time data updates. This is quite an interesting deep dive from Uber into their challenges and lessons learned throughout their large-scale data journey. |
|
---|
|
Microsoft's CPU Inference ML Lib Microsoft has released an exciting project to bring GPU-native LLMs into CPUs with Bitnet.cpp; this is their official inference framework for 1-bit Large Language Models: This C++ library is inspired from Llama.cpp and provides optimized kernels for fast and energy-efficient inference on CPUs, with future support planned for NPUs and GPUs. This framework achieves significant speedups of 6×+ and energy reductions up to 82.2% on both ARM and x86 architectures, which is quite exciting for even 100B-parameter models. |
|
|
---|
|
Meta's Open AI Data & Models Meta's FAIR team has released several new AI research artifacts to continue supporting the advancement of science across the community: These open source releases include: SAM 2.1 dataset on image and video segmentation; Meta Spirit LM as multimodal language model integrating speech and text; Layer Skip to accelerate LLM performance; SALSA to validate security for post-quantum cryptography standards; Meta Lingua for large-scale language model training; Meta Open Materials 2024 to accelerate AI-assisted inorganic materials discovery, and more. |
|
|
---|
|
Upcoming MLOps Events The MLOps ecosystem continues to grow at break-neck speeds, making it ever harder for us as practitioners to stay up to date with relevant developments. A fantsatic way to keep on-top of relevant resources is through the great community and events that the MLOps and Production ML ecosystem offers. This is the reason why we have started curating a list of upcoming events in the space, which are outlined below. Upcoming conferences where we're speaking: Other upcoming MLOps conferences in 2024:
In case you missed our talks:
|
|
---|
| |
Check out the fast-growing ecosystem of production ML tools & frameworks at the github repository which has reached over 10,000 ⭐ github stars. We are currently looking for more libraries to add - if you know of any that are not listed, please let us know or feel free to add a PR. Four featured libraries in the GPU acceleration space are outlined below. - Kompute - Blazing fast, lightweight and mobile phone-enabled GPU compute framework optimized for advanced data processing usecases.
- CuPy - An implementation of NumPy-compatible multi-dimensional array on CUDA. CuPy consists of the core multi-dimensional array class, cupy.ndarray, and many functions on it.
- Jax - Composable transformations of Python+NumPy programs: differentiate, vectorize, JIT to GPU/TPU, and more
- CuDF - Built based on the Apache Arrow columnar memory format, cuDF is a GPU DataFrame library for loading, joining, aggregating, filtering, and otherwise manipulating data.
If you know of any open source and open community events that are not listed do give us a heads up so we can add them! |
|
---|
| |
As AI systems become more prevalent in society, we face bigger and tougher societal challenges. We have seen a large number of resources that aim to takle these challenges in the form of AI Guidelines, Principles, Ethics Frameworks, etc, however there are so many resources it is hard to navigate. Because of this we started an Open Source initiative that aims to map the ecosystem to make it simpler to navigate. You can find multiple principles in the repo - some examples include the following: - MLSecOps Top 10 Vulnerabilities - This is an initiative that aims to further the field of machine learning security by identifying the top 10 most common vulnerabiliites in the machine learning lifecycle as well as best practices.
- AI & Machine Learning 8 principles for Responsible ML - The Institute for Ethical AI & Machine Learning has put together 8 principles for responsible machine learning that are to be adopted by individuals and delivery teams designing, building and operating machine learning systems.
- An Evaluation of Guidelines - The Ethics of Ethics; A research paper that analyses multiple Ethics principles.
- ACM's Code of Ethics and Professional Conduct - This is the code of ethics that has been put together in 1992 by the Association for Computer Machinery and updated in 2018.
If you know of any guidelines that are not in the "Awesome AI Guidelines" list, please do give us a heads up or feel free to add a pull request!
|
|
---|
| |
| | The Institute for Ethical AI & Machine Learning is a European research centre that carries out world-class research into responsible machine learning. | | |
|
|
---|
|
|
This email was sent to You received this email because you are registered with The Institute for Ethical AI & Machine Learning's newsletter "The Machine Learning Engineer" |
| | |
|
|
---|
|
© 2023 The Institute for Ethical AI & Machine Learning |
|
---|
|
|
|